Transforming Healthcare: The Impact of Instance Segmentation on Medical Imaging and Patient Care
Instance segmentation, a branch of computer vision, has emerged as a game-changer in the medical field. Unlike traditional segmentation methods that identify only the general presence of a structure, instance segmentation goes a step further. It pinpoints and delineates individual objects within a medical image, offering a more granular level of analysis with significant downstream impacts.
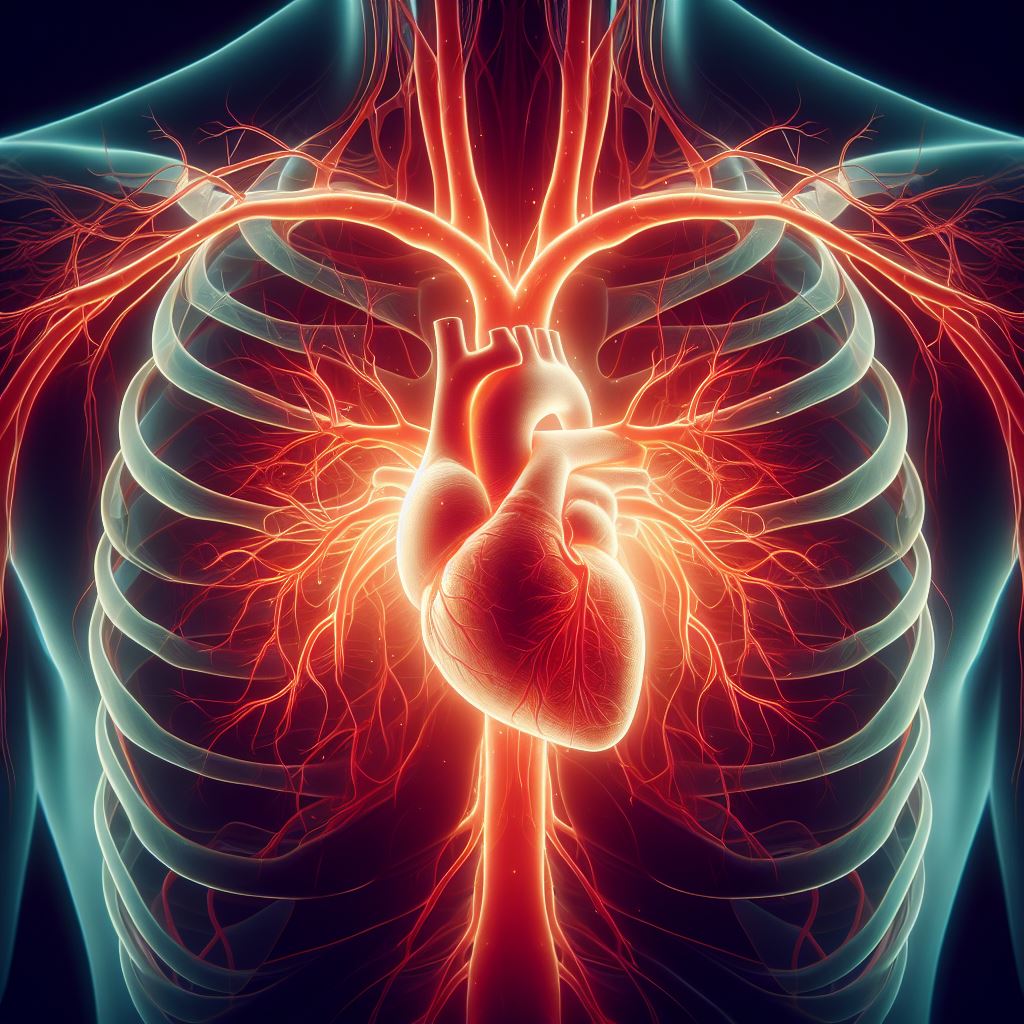
Introduction to Instance Segmentation in the Medical Field
Instance segmentation, a computer vision technique that identifies and delineates individual objects within an image, has revolutionized numerous industries, including healthcare. In the medical field, where accurate and precise analysis of imaging data is crucial for diagnosis, treatment, and research, instance segmentation offers unparalleled opportunities for enhancing medical imaging techniques, improving patient care, and advancing scientific understanding. This article explores the profound impact of instance segmentation on various aspects of the medical field, from diagnostics to therapeutics.
Enhanced Medical Imaging Analysis
One of the most significant impacts of instance segmentation in the medical field is its ability to enhance the analysis of medical imaging data. By precisely delineating individual structures and abnormalities within images such as MRI scans, CT scans, and X-rays, instance segmentation algorithms provide clinicians with detailed insights into anatomical features, lesions, tumors, and other pathological conditions. This enables more accurate diagnosis, treatment planning, and monitoring of patients, ultimately leading to improved outcomes.
Automated Disease Detection and Diagnosis
Instance segmentation plays a pivotal role in automating disease detection and diagnosis processes. By accurately identifying and segmenting specific abnormalities within medical images, such as tumors, nodules, or lesions, machine learning models powered by instance segmentation can assist radiologists and clinicians in rapidly screening large volumes of imaging data. This not only reduces the burden on healthcare professionals but also enables earlier detection and intervention for various medical conditions, potentially saving lives and improving prognosis.
Precision Medicine and Personalized Treatment
The application of instance segmentation in the medical field facilitates precision medicine and personalized treatment approaches. By precisely delineating individual structures and pathologies within medical images, clinicians can tailor treatment strategies to each patient’s unique anatomy and disease characteristics. This enables more targeted interventions, such as precise surgical resections or radiation therapy targeting specific tumor volumes, while minimizing damage to surrounding healthy tissues. Additionally, instance segmentation aids in identifying biomarkers and genetic signatures associated with certain diseases, enabling the development of targeted therapies and predictive models for patient outcomes.
Advancements in Surgical Navigation and Intervention
Instance segmentation has revolutionized surgical navigation and intervention by providing surgeons with detailed, real-time guidance during procedures. By overlaying segmented structures and abnormalities onto surgical field images, augmented reality systems powered by instance segmentation enable surgeons to precisely localize and navigate around critical anatomical structures, enhancing surgical accuracy and reducing complications. This technology is particularly valuable in complex procedures such as neurosurgery, where precise localization and navigation are paramount for successful outcomes.
Facilitation of Medical Research and Education
In addition to its clinical applications, instance segmentation facilitates medical research and education by providing researchers and educators with powerful tools for analyzing and visualizing medical imaging data. By accurately segmenting anatomical structures and pathologies, researchers can gain deeper insights into disease mechanisms, treatment responses, and patient outcomes. Moreover, visual software that use instance segmentations in their output enable the creation of interactive educational resources and simulations that enhance medical training and enable students to practice interpreting complex imaging studies in a simulated environment.
Future Directions and Opportunities
The future of instance segmentation in medicine is brimming with exciting possibilities. Research will focus on refining the technology to achieve even greater accuracy, efficiency, and interpretability. This can be accomplished by incorporating data from multiple imaging sources (X-ray, MRI, etc.) to create a more comprehensive picture, utilizing cutting-edge machine learning techniques, and infusing the algorithms with the deep knowledge base of medical professionals. Furthermore, the development of interoperable and scalable solutions is crucial. Imagine instance segmentation tools that seamlessly integrate with existing clinical workflows and electronic health record systems, allowing for a smooth transition into daily practice. By overcoming these hurdles, instance segmentation unlocks the door to a new era of precision medicine. Physicians will be empowered with a powerful tool for identifying and analyzing specific structures within medical images, leading to more accurate diagnoses, personalized treatment plans, and ultimately, improved patient outcomes. The potential for earlier disease detection, more effective therapies, and better overall patient care makes instance segmentation a transformative technology with the potential to reshape the landscape of medicine.
In conclusion, instance segmentation stands as a pivotal advancement in the medical field, offering unprecedented opportunities to revolutionize healthcare delivery. Its ability to precisely identify and delineate individual objects within medical images has profoundly impacted various aspects of medical practice, from diagnostics to therapeutics. By enhancing medical imaging analysis, automating disease detection and diagnosis, and facilitating precision medicine and personalized treatment, instance segmentation has significantly improved patient care and outcomes. Furthermore, its applications in surgical navigation and intervention, as well as medical research and education, highlight its versatility and potential to transform healthcare delivery and medical education. Looking ahead, the future of instance segmentation in medicine holds promise for even greater advancements. Continued research and development efforts will focus on refining the technology to achieve higher levels of accuracy, efficiency, and interpretability. The integration of instance segmentation into existing clinical workflows and electronic health record systems will further enhance its utility and impact. Ultimately, instance segmentation represents a transformative technology poised to usher in a new era of precision medicine, personalized care, and improved patient outcomes. Its potential to enable earlier disease detection, more effective therapies, and better overall patient care underscores its significance in reshaping the landscape of medicine for the better.
References
Xiong, J., Nguyen, E. H., Liu, Y., Deng, R., Tyree, R. N., Correa, H., … & Huo, Y. (2024). Circle Representation for Medical Instance Object Segmentation. arXiv preprint arXiv:2403.11507.
Dhanakshirur, R. R., Shastry, K. A., Borgavi, K., Suri, A., Kalra, P. K., & Arora, C. (2023, October). Learnable query initialization for surgical instrument instance segmentation. In International Conference on Medical Image Computing and Computer-Assisted Intervention (pp. 728-738). Cham: Springer Nature Switzerland.
Nasir, E. S., Parvaiz, A., & Fraz, M. M. (2023). Nuclei and glands instance segmentation in histology images: a narrative review. Artificial Intelligence Review, 56(8), 7909-7964.
Pan, H., Zhang, M., Bai, W., Li, B., Wang, H., Geng, H., … & Chen, M. (2023). An instance segmentation model based on deep learning for intelligent diagnosis of uterine myomas in mri. Diagnostics, 13(9), 1525.